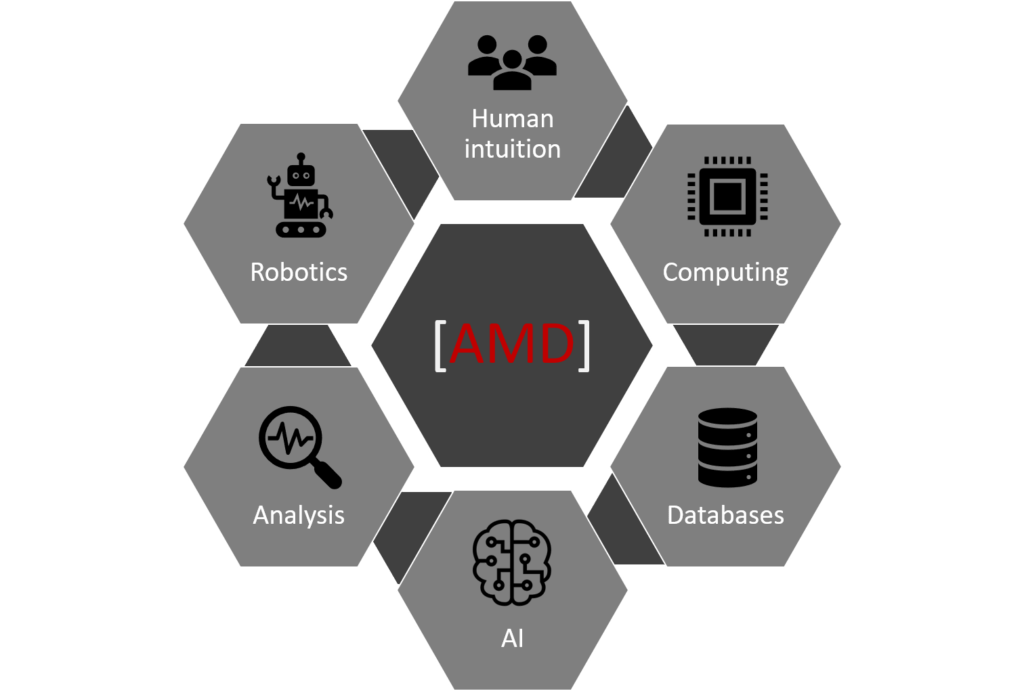
At the Autonomous Energy Materials Discovery [AMD] research group our focus is on developing and using predictive methods for the discovery of molecules and materials for energy applications, which is fully in line with DIFFER’s mission.
Our research endeavors can be categorized into the following five groups:
1) Physics-based calculations: We develop and use high-throughput computational screening workflows for the classical and quantum chemical modelling of compounds at the nanoscale. This way we generate trustworthy computational (i.e. synthetic) data on chemical compounds, which is an add-on to existing molecule and material databases.
2) Molecule/material databases: FAIR data is the foundation of AI. To improve AI-aided design of molecules and materials, we locally generate or collect, merge, organize, and label the existing datasets in a unifying manner towards a generalizable approach.
3) Discriminative AI models: For the forward design of molecules and materials, we use various data-driven AI models and their hybrid forms to draw reliable predictions of properties directly from line notation representation of molecules and materials. We integrate useful data into refined models and ontologies and execute them in low-data environments, thereby improving the accuracy of predictions.
4) Generative AI models: For the reverse design of materials, we use deep generative models with an aim to enlarge the applicability domains of the models. This way we can design completely new molecules and materials that have not been considered before for the target energy storage or conversion application.
5) Research software: We develop software tools, such as AI-powered molecule and material property prediction tools, and high-dimensional data visualization for molecule discovery and explainable AI. These tools are an integral piece of our work, including diversity analysis, outlier detection, virtual chemical library design, screening, and the follow-up engineering of compounds.
In recent years we have been working on: a) Energy storage molecules, primarily for redox flow battery electrolytes and Li/Na-ion battery cathodes, and b) Energy conversion materials, including 2D materials and ABO3 metal-oxides for the conversion of H2O, CO2, and N2 into H2, CO, and NH3, respectively.